Unveiling the Future: A Deep Dive into Artificial Intelligence
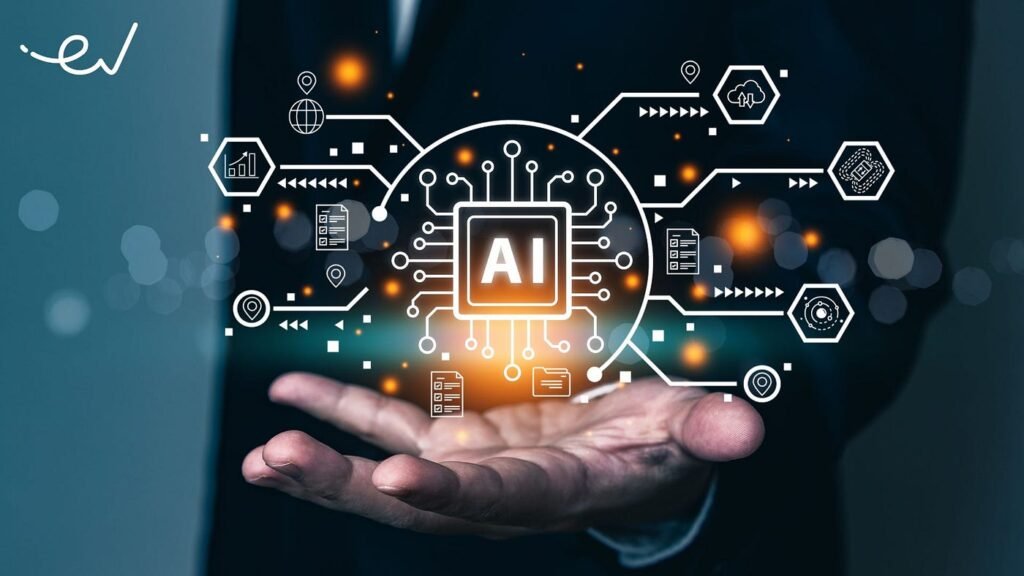
Applications Across Industries:
In healthcare, the application of AI goes beyond diagnostic tools. AI-powered predictive analytics help healthcare providers anticipate patient needs, optimize resource allocation, and improve operational efficiency. Natural language processing (NLP) algorithms analyze unstructured medical records, extracting valuable insights for clinical decision-making and research. Virtual health assistants powered by AI algorithms offer personalized health recommendations, medication reminders, and support for patients managing chronic conditions.
In finance, AI algorithms drive algorithmic trading, risk management, and fraud detection. High-frequency trading systems execute trades in milliseconds, leveraging machine learning models to analyze market data and identify profitable opportunities. AI-powered chatbots provide personalized financial advice, answer customer inquiries, and streamline customer service operations. Risk assessment models powered by AI algorithms evaluate creditworthiness, insurance claims, and investment portfolios, enabling financial institutions to make data-driven decisions and mitigate risks.
In manufacturing, AI-driven robotics and automation systems revolutionize production processes, enabling agile, flexible, and adaptive manufacturing environments. Collaborative robots (cobots) work alongside human workers, performing intricate tasks with precision and efficiency. AI-powered predictive maintenance systems monitor equipment health in real-time, detecting anomalies and scheduling maintenance interventions to prevent unplanned downtime and costly production delays. Supply chain optimization algorithms powered by AI analyze demand forecasts, inventory levels, and transportation logistics to streamline operations and reduce costs.
Across transportation, AI plays a pivotal role in autonomous vehicles, intelligent traffic management systems, and smart infrastructure solutions. Self-driving cars equipped with AI algorithms navigate roads with human-like perception and decision-making capabilities, leveraging sensor fusion, computer vision, and machine learning to detect and respond to dynamic traffic conditions. AI-powered traffic management systems optimize traffic flow, minimize congestion, and reduce emissions by coordinating signal timings and routing decisions in real-time. Autonomous drones powered by AI algorithms enable last-mile delivery services, aerial inspections, and disaster response operations, offering cost-effective and scalable solutions for various applications.
Ethical Considerations:
Algorithmic bias poses a significant ethical challenge in AI development and deployment, perpetuating existing inequalities and reinforcing systemic biases present in training data. Fairness-aware algorithms aim to mitigate bias by promoting equity, transparency, and accountability in AI decision-making processes. Techniques such as fairness constraints, bias detection, and bias mitigation algorithms help developers identify and address bias in AI systems, ensuring that outcomes are fair and equitable for all individuals, regardless of race, gender, or socioeconomic status.
Data privacy concerns arise from the proliferation of personal data collected by AI systems, raising questions about consent, transparency, and user control over data usage. Privacy-preserving AI techniques, such as federated learning, differential privacy, and homomorphic encryption, enable collaborative model training while protecting sensitive data from unauthorized access or misuse. Privacy-enhancing technologies empower individuals to retain ownership and control over their data, fostering trust and accountability in AI-driven applications and services.
The rise of autonomous weapons and lethal autonomous systems (LAS) raises profound ethical questions about the morality and legality of delegating life-and-death decisions to machines. The Campaign to Stop Killer Robots advocates for an international ban on fully autonomous weapons systems that lack human oversight and accountability, citing concerns about compliance with international humanitarian law, ethical implications, and the risk of unintended consequences. Multistakeholder dialogues and diplomatic efforts are essential for addressing these ethical challenges and establishing norms, regulations, and safeguards to prevent the proliferation of autonomous weapons and protect human rights in armed conflict.
Challenges and Limitations:
The curse of dimensionality poses a fundamental challenge in AI, particularly for high-dimensional data spaces where traditional machine learning algorithms struggle to generalize effectively. Techniques such as dimensionality reduction, feature engineering, and model regularization help mitigate the curse of dimensionality by simplifying complex data representations and improving the generalization performance of AI models. However, scaling AI algorithms to handle massive datasets and high-dimensional feature spaces remains an ongoing research challenge that requires interdisciplinary collaboration and innovative solutions.
The scarcity of labeled data presents a significant bottleneck in AI development, as supervised learning algorithms rely on large amounts of annotated data to train accurate predictive models. Weakly supervised learning, semi-supervised learning, and self-supervised learning techniques offer alternative approaches for leveraging unlabeled or partially labeled data to train AI models without requiring extensive manual annotation. Active learning strategies, data augmentation techniques, and transfer learning methods help alleviate the need for large labeled datasets by leveraging existing knowledge and domain expertise to bootstrap model training and adaptation processes.
The quest for artificial general intelligence (AGI), or human-level AI, remains a grand challenge in AI research, with researchers grappling with fundamental questions about consciousness, creativity, and common sense reasoning. Building AGI requires interdisciplinary collaboration, breakthroughs in cognitive science, and a deep understanding of human cognition and intelligence. OpenAI’s GPT-3 model represents a significant milestone in natural language understanding and generation, demonstrating remarkable capabilities in text-based tasks such as language translation, question answering, and content generation. However, achieving AGI requires advances in perception, reasoning, and decision-making across diverse modalities and domains, posing complex technical and philosophical challenges that transcend the capabilities of current AI systems.
Future Horizons:
Looking ahead, the future of AI is brimming with promise and potential, fueled by advancements in research, technology, and interdisciplinary collaboration. Explainable AI (XAI) aims to demystify AI decision-making processes, enabling humans to understand, trust, and interpret AI predictions and recommendations. Techniques such as attention mechanisms, interpretability methods, and model-agnostic approaches help elucidate the inner workings of AI algorithms and provide insights into their decision-making rationale.
Federated learning represents a decentralized approach to AI model training, where data remains localized on device or edge devices, preserving privacy and security while enabling collaborative model updates across distributed networks. Federated learning holds immense potential for applications in healthcare, finance, and IoT, where data privacy and regulatory compliance are paramount. Techniques such as federated averaging, differential privacy, and secure aggregation enable collaborative model training while protecting sensitive data from unauthorized access or disclosure.
Quantum computing represents a paradigm shift in computing power, offering exponential speedups for AI tasks such as optimization, simulation, and cryptography. Quantum machine learning algorithms leverage quantum phenomena such as superposition and entanglement to solve complex problems beyond the reach of classical computers. Google’s quantum supremacy experiment demonstrated the potential of quantum computers to outperform classical computers on specific tasks, sparking renewed interest and investment in quantum computing research and development. However, realizing the full potential of quantum computing for AI requires overcoming technical challenges such as qubit coherence, error correction, and scalability, as well as exploring new algorithmic paradigms and applications tailored to quantum hardware architectures.
AI ethics and governance frameworks are essential for ensuring that AI technologies are developed, deployed, and used in a manner that aligns with societal values, human rights, and ethical principles. Multistakeholder collaborations involving governments, industry, academia, and civil society are crucial for shaping AI policies, standards, and guidelines that promote innovation, transparency, and accountability while mitigating risks and safeguarding human welfare. Initiatives such as the Partnership on AI, the Montreal Declaration for Responsible AI, and the European Union’s AI Ethics Guidelines provide frameworks for ethical AI development, deployment, and regulation, emphasizing principles such as fairness, transparency, accountability, and human-centricity.
Empowering Humanity:
Ultimately, the true measure of AI’s success lies not just in its technological prowess but in its capacity to empower humanity. By embracing AI with humility, foresight, and a steadfast commitment to ethical principles, we can harness its transformative power to tackle pressing global challenges, enhance human capabilities, and foster inclusive societies where innovation thrives, humanity flourishes, and the boundaries of possibility are endlessly redrawn. From healthcare and finance to manufacturing and transportation, AI empowers individuals and organizations to unleash their creativity, ingenuity, and resilience, shaping a future where technology serves as a force for good, enriching lives, and advancing human flourishing.